# About Testin
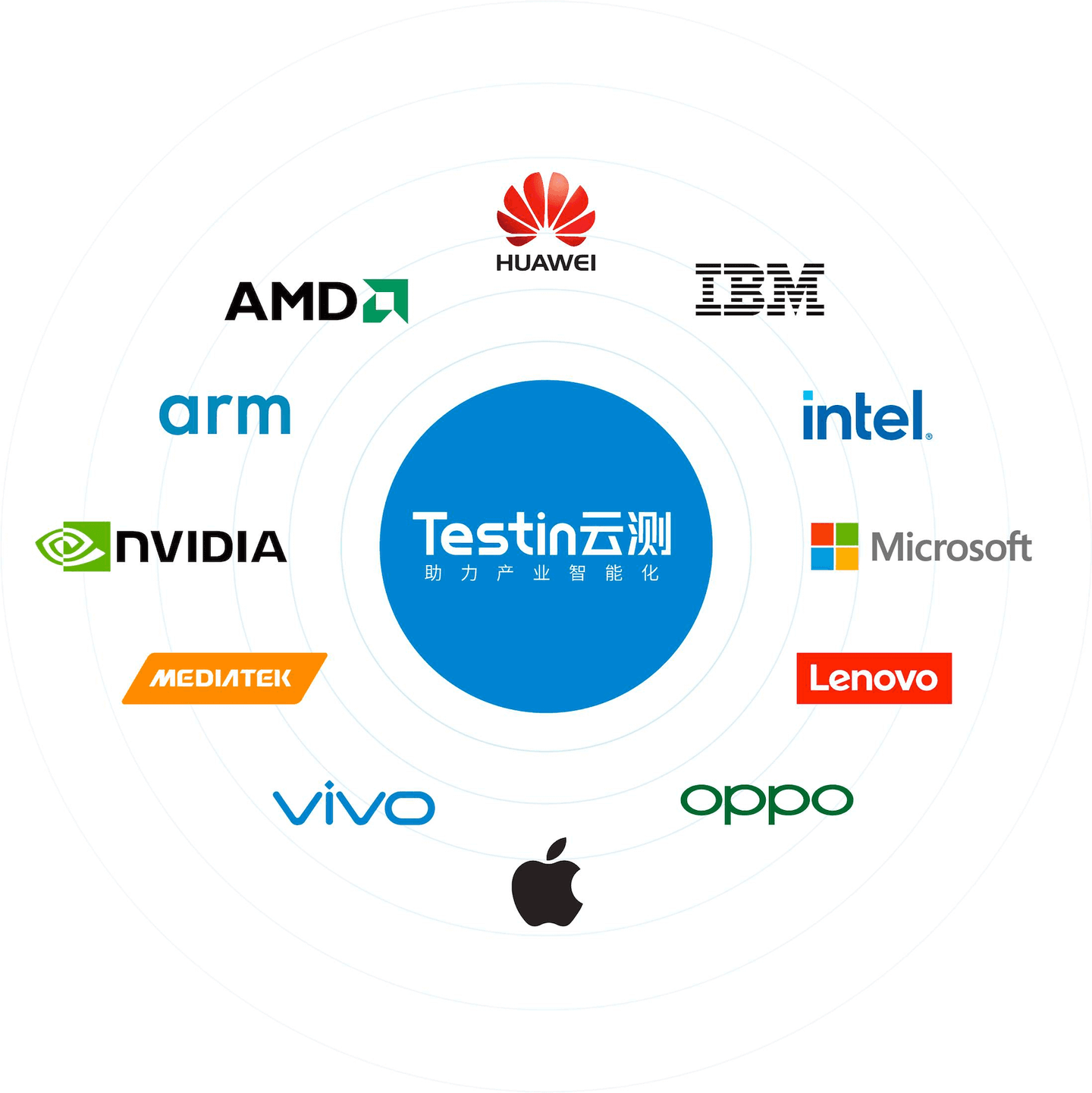
Founded in 2011, Testin is an enterprise service platform that provides two main services: a cloud testing service for developers, which has served over 800,000 developers and performed more than 150 million tests for 2 million apps, and a machine learning (ML) model training service that includes services such as data annotation and model deployment in industries such as security and the Internet of Things (IoT). To date, Testin has collaborated with over 110 companies, ranging from car manufacturers offering self-driving autonomous technology to smart home and finance firms dealing with facial recognition and natural language processing applications. Testin has also received several prestigious awards in China, including Deloitte China's top 50 high-tech companies and Red Herring Global Top 100.
# Prior to using MyScale
MyScale collaborated with Testin to find new ways to make the AI data annotation service as useful as possible. A typical Testin AI data annotation platform user will go through the following steps:
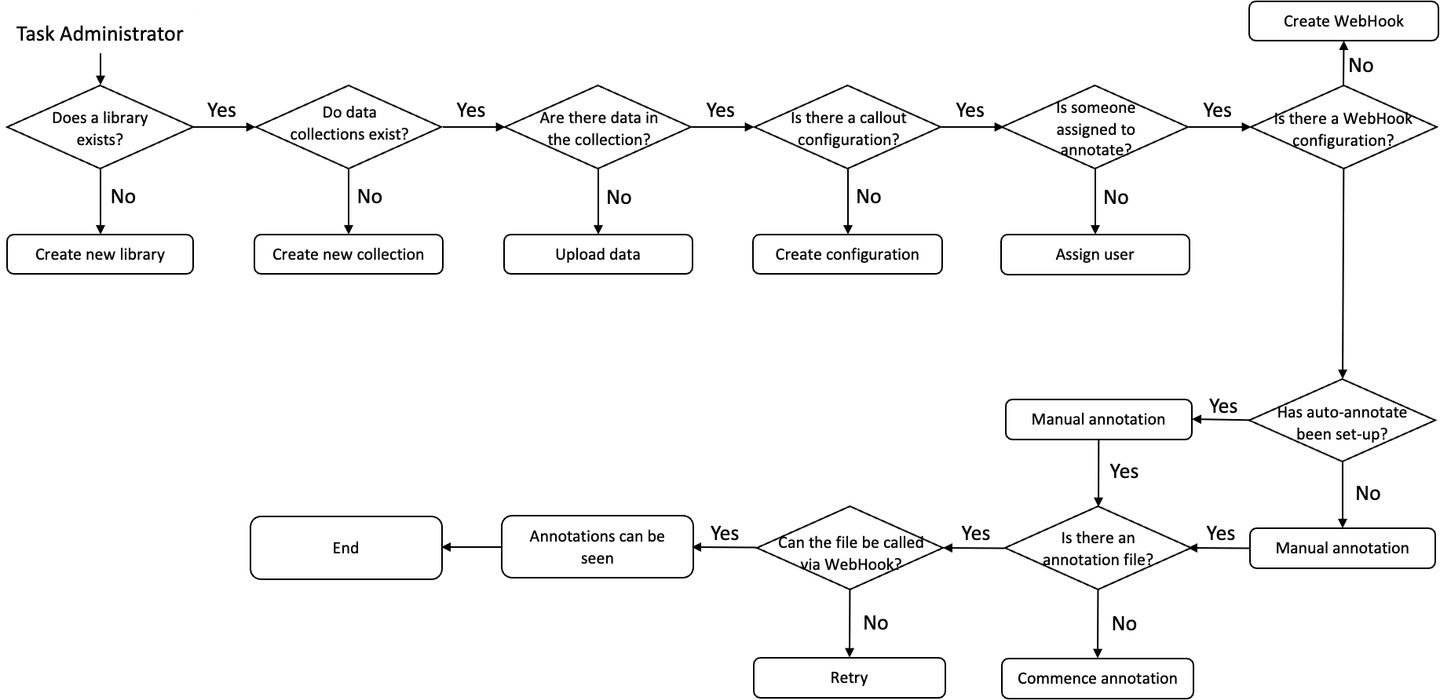
A user first creates a collection within a library. After that, data is added to the collection before annotation of the data can begin. The AI data annotation platform then exports the data for use in other applications, such as machine learning models.
While the process of data annotation may appear simple, it can be time-consuming. For example, while Testin's auto-annotate feature can help users save time on annotation, it is not always reliable. As seen in the image, the platform could only identify one car and could not identify other cars or larger vehicles, such as a bus. Because of the lack of consistent, high-quality annotations across all images, only a small number of images have accurate annotations.
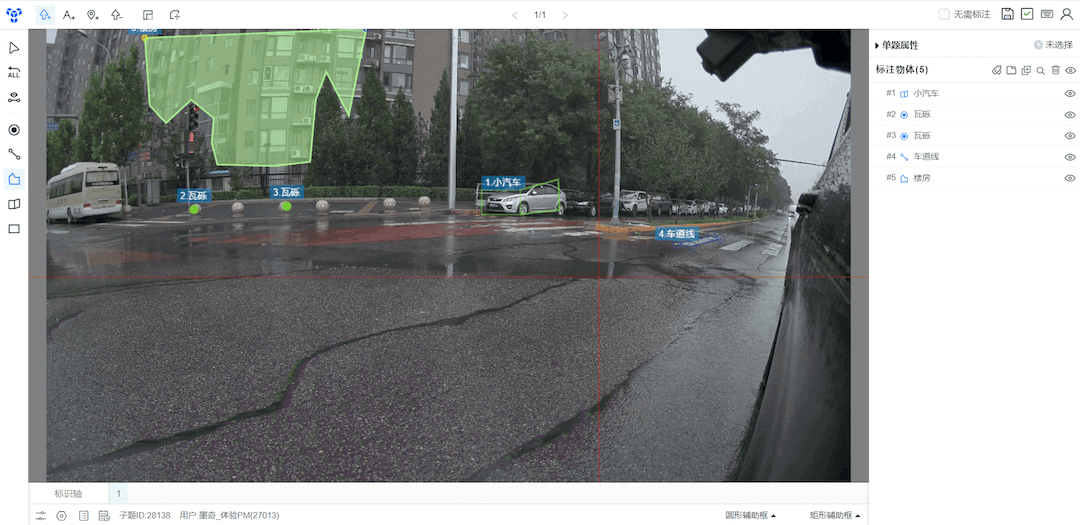
On the other hand, if a user wishes to annotate, it would be time-consuming, though the quality of the annotations would be improved. Throughout this process, a user must manually identify and categorize the areas of an image that are relevant to the self-driving car. However, for most users, manually annotating a large batch of images is impractical. As a result, users are often required to choose from the auto-annotated photos and improve the quality of the annotations.
Another issue with the current process was that the data and their annotations could not be transferred seamlessly from one platform to another. Users of the Testin AI data platform currently do not have the option of storing all of their annotations on a cloud drive of their choice that allows them to access them. They must download the annotations to their local drives before they can execute similarity searches or train their AI models.
# Leveraging MyScale’s vector search functions on Testin AI data platform
As such, MyScale proposed that Testin consider two changes to their AI data platform:
Leveraging MyScale’s unsupervised learning feature for small samples
Testin users to execute joint queries on the MyScale platform from both on-premise and cloud devices.
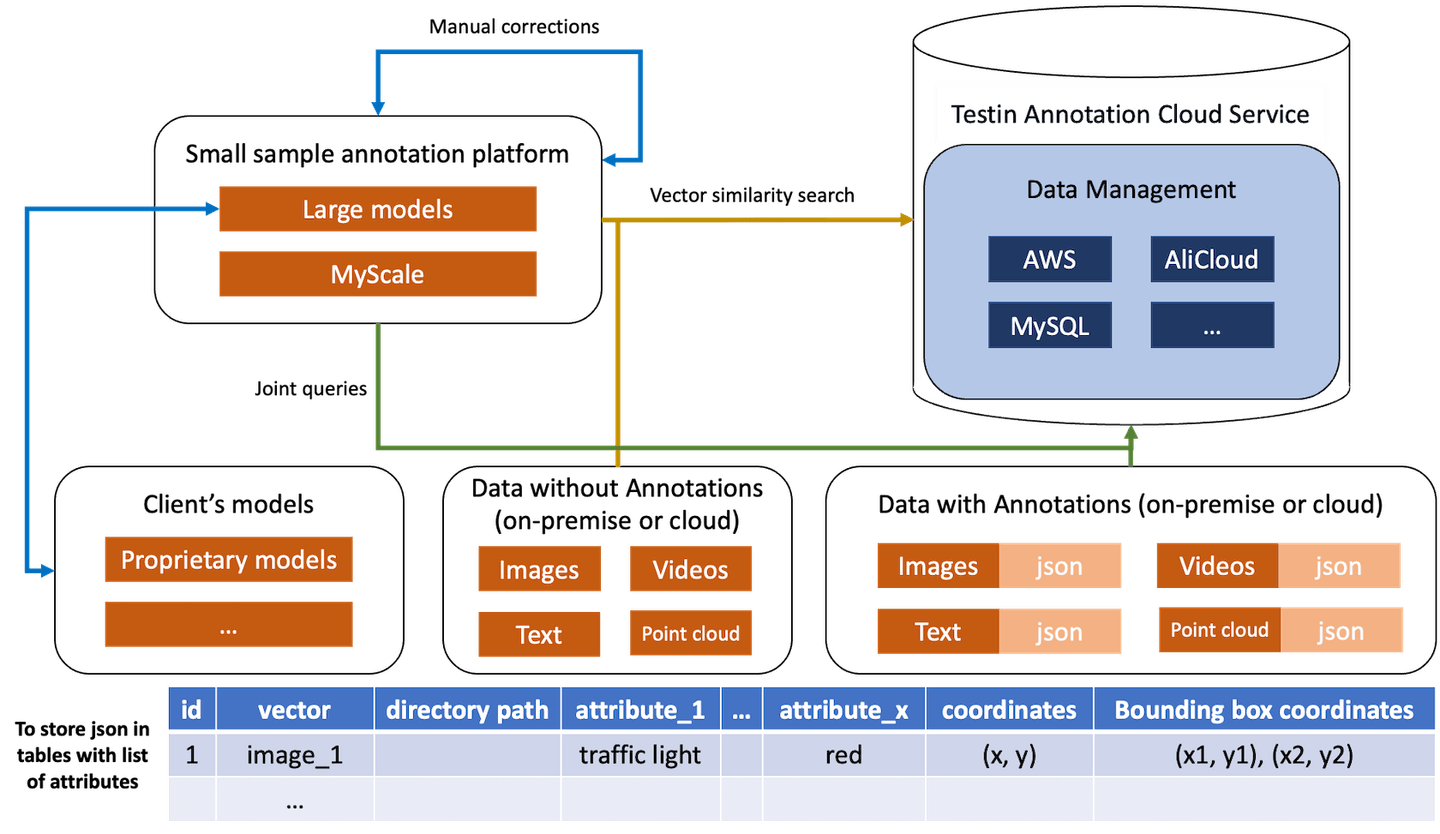
For the former, MyScale allows users to select specific images from the batches of images with or without annotations to determine if they require additional work on their annotations. This meant that a user who used Testin’s auto-annotate feature could use MyScale to quickly search through the batch of annotations and identify which images had good/poor (or no) annotations, rather than manually inspecting for images and determining if the batches of images had good matches to a good quality sample photo with annotations. This is also useful when annotating a repository of photos that is constantly being updated with new images and data, as using MyScale eliminates the need for users to sift through the images to determine if annotations are required.
For the latter, users can use the MyScale platform’s SQL query feature to perform vector similarity searches from both their linked cloud database accounts and the vector files they provided locally to match against a query vector. For example, if the query is a photo or text, MyScale will search for similar text and photos, as well as similar videos and other data modalities. Furthermore, when two vectors of high similarity were discovered, Testin used this feature to filter out duplicate data.
# How MyScale can help you in unlocking new business value with vector search
MyScale collaborated with the software testing firm Testin to improve their data labeling workflow. Testin used MyScale’s SQL and high-performing vector search capabilities to build their own automatic data annotation and data de-duplication functions. While manually annotating data is common, it is also a bottleneck in ensuring high-quality data annotations, as well as time and cost performance. MyScale assisted Testin in developing automatic data annotation and data de-duplication functions using MyScale’s SQL functions.
As a high performing database suited to the development of machine learning models and applications, Testin improved its data annotation workflow by automating processes and reducing annotation time, storage space, and cost.
If your business is also dealing with cloud storages in your current application, and would like to explore more on how MyScale can help extract more value from your applications and businesses, please don't hesitate to contact us at contact@myscale.com.