# 引言
强大的语言模型如GPT3.5-turbo和GPT4已经彻底改变了应用开发,导致了领域特定应用的激增。像PandaGPT和GiftWarp这样的杰出应用展示了这些模型的能力。这些应用之所以与众不同,是因为它们在数据处理方面表现出色。例如,PandaGPT可以无缝地从数百个PDF文档中检索信息。这使得PandaGPT在竞争激烈的应用市场中取得成功。
为了确保应用的长久发展,创业者必须优先考虑数据处理的扩展性。随着应用的普及,高效的数据处理变得至关重要。强大的基础设施和可扩展的系统对于管理增加的数据负载至关重要。通过解决瓶颈问题并规划平稳扩展,创业者可以使他们的应用实现增长并满足用户需求。
采用数据驱动的方法提供了前所未有的机会。借助GPT3.5-turbo和GPT4等语言模型,开发人员可以释放创新和卓越用户体验的潜力。通过利用这些模型的力量,应用开发达到了新的高度。未来的发展在于数据驱动的解决方案,并利用先进的语言模型实现变革性体验。
# 从一开始就规划可扩展性
借助OpenAI的API,我们可以利用GPT和少量产品数据轻松创建一个客户服务聊天机器人。通过使用GPT分析提示,我们可以高效地在给定列表中搜索项目并取得令人印象深刻的结果。以下是一个示例:
import os
import openai
from dotenv import load_dotenv, find_dotenv
_ = load_dotenv(find_dotenv()) # 读取本地的.env文件
openai.api_key = os.environ['OPENAI_API_KEY']
delimiter = "####"
system_message = f"""
Follow these steps to answer the customer queries.
The customer query will be delimited with four hashtags,
i.e. {delimiter}.
Step 1:{delimiter} First decide whether the user is
asking a question about a specific product or products.
Product cateogry doesn't count.
Step 2:{delimiter} If the user is asking about
specific products, identify whether
the products are in the following list.
All available products:
1. Product: TechPro Ultrabook
Category: Computers and Laptops
Brand: TechPro
Model Number: TP-UB100
Warranty: 1 year
Rating: 4.5
Features: 13.3-inch display, 8GB RAM, 256GB SSD, Intel Core i5 processor
Description: A sleek and lightweight ultrabook for everyday use.
Price: $799.99
2. Product: BlueWave Gaming Laptop
Category: Computers and Laptops
Brand: BlueWave
Model Number: BW-GL200
Warranty: 2 years
Rating: 4.7
Features: 15.6-inch display, 16GB RAM, 512GB SSD, NVIDIA GeForce RTX 3060
Description: A high-performance gaming laptop for an immersive experience.
Price: $1199.99
3. Product: PowerLite Convertible
Category: Computers and Laptops
Brand: PowerLite
Model Number: PL-CV300
Warranty: 1 year
Rating: 4.3
Features: 14-inch touchscreen, 8GB RAM, 256GB SSD, 360-degree hinge
Description: A versatile convertible laptop with a responsive touchscreen.
Price: $699.99
......
Step 3:{delimiter} If the message contains products
in the list above, list any assumptions that the
user is making in their
message e.g. that Laptop X is bigger than
Laptop Y, or that Laptop Z has a 2 year warranty.
Step 4:{delimiter}: If the user made any assumptions,
figure out whether the assumption is true based on your
product information.
Step 5:{delimiter}: First, politely correct the
customer's incorrect assumptions if applicable.
Only mention or reference products in the list of
5 available products, as these are the only 5
products that the store sells.
Answer the customer in a friendly tone.
Use the following format:
Step 1:{delimiter} <step 1 reasoning>
Step 2:{delimiter} <step 2 reasoning>
Step 3:{delimiter} <step 3 reasoning>
Step 4:{delimiter} <step 4 reasoning>
Response to user:{delimiter} <response to customer>
Make sure to include {delimiter} to separate every step.
"""
user_message = f"""
how much is the BlueWave Chromebook more expensive
than the TechPro Desktop"""
messages = [
{'role':'system',
'content': system_message},
{'role':'user',
'content': f"{delimiter}{user_message}{delimiter}"},
]
response = get_completion_from_messages(messages)
print(response)
如果一家初创公司旨在开发一个结合多模态功能并利用更大数据集的客户服务聊天机器人,那么在这个阶段使用向量数据库是必要的,以满足他们的需求。在这个目标的实现过程中,使用一个专门设计用于处理高维数据(包括多模态信息)的向量数据库至关重要。借助向量数据库,我们可以存储和索引表示客户服务数据不同方面的向量,例如文本、图像甚至音频。
例如,当客户向聊天机器人提交查询时,系统可以使用自然语言处理技术将文本转换为向量表示。然后,可以使用这个向量表示在向量数据库中搜索相关的响应。此外,如果聊天机器人能够处理图像或音频输入,这些输入也可以转换为向量表示并存储在数据库中。
向量数据库高效地索引这些向量,实现快速检索相关信息的功能。通过利用最近邻搜索等先进的搜索算法,聊天机器人可以根据用户查询与数据库中存储的向量之间的相似度指标识别最合适的响应。
随着数据集的扩大,向量数据库确保了多模态数据的可扩展性和高效存储。它简化了更新和添加数据集的过程,使聊天机器人能够不断改进性能,并对客户查询提供准确和相关的响应。
# 在可扩展性和成本效益之间取得平衡
在实现可扩展性的同时,初创公司还需要考虑成本效益。通过结构化和预处理数据以提取相关特征和属性,可以减少存储和处理需求,从而降低成本。利用提供多模态功能的现有工具和框架也可以节省宝贵的时间和资源。这些资源通常具有优化的数据结构和算法,无需从头开始构建一切。
在选择数据库时,初创公司应考虑MyScale作为一种具有成本效益的解决方案。MyScale是一种密集且成本效益高的向量数据库,与其他替代方案相比,提供了更高的性能。通过实施结构化和预处理数据的策略,利用现有的工具和框架,并考虑MyScale等成本效益高的解决方案,初创公司可以在可扩展性和成本效益之间取得平衡。这些方法在最大程度利用可用资源的同时优化性能,使初创公司能够以成本效益的方式实现增长和成功。
# 案例研究和最佳实践
在这里,我们将简要介绍如何使用MyScale快速扩展多模态客户服务聊天机器人。为此,我们使用了从淘宝直播 (opens new window)中提取的简化数据集。
# 安装前提条件
transformers
:运行CLIP模型tqdm
:人性化的美观进度条clickhouse-connect
:MyScale数据库客户端
python3 -m pip install transformers tqdm clickhouse-connect streamlit pandas lmdb torch
# 进入数据
首先,让我们来看一下数据集的结构,我们将数据分成了两个表格。
id | product_url | label |
---|---|---|
102946 | url_to_store_the image | Men's Long Sleeve Shirt |
数据集包含三列:产品ID、产品图像和标签。第一列用作每个产品图像的唯一标识符。第二列包含指向图像的URL。第三列是产品的标签。以下是上述提到的product_url列的示例:
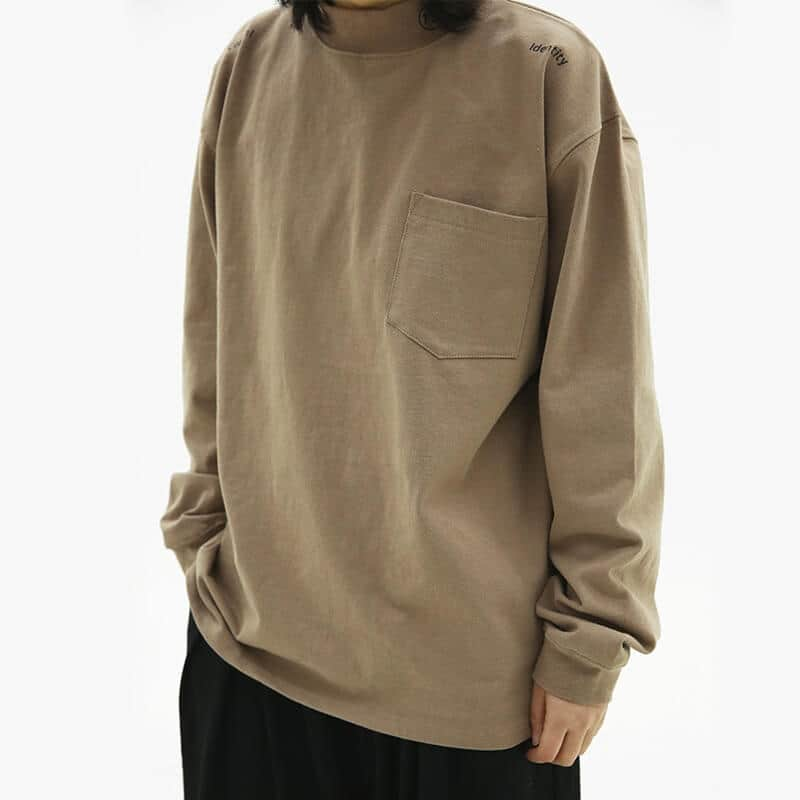
id | product_text | label |
---|---|---|
102946 | POOF C(1's)I MOCK NECK POCKET TEE | Men's Long Sleeve Shirt |
# 创建MyScale数据库表格
# 使用数据库
您需要与数据库后端建立连接,以在MyScale中创建表格。您可以在此页面 (opens new window)上查看有关Python客户端的详细指南。
如果您熟悉SQL(结构化查询语言),那么在MyScale中使用起来会更加容易。MyScale将结构化查询与向量搜索相结合,这意味着创建向量数据库几乎与创建传统数据库相同。以下是我们在SQL中创建两个向量表格的示例:
CREATE TABLE IF NOT EXISTS TaoBaoData_image(
id String,
vector Array(Float32),
CONSTRAINT vec_len CHECK length(vector) = 512
) ENGINE = MergeTree ORDER BY id;
CREATE TABLE IF NOT EXISTS TaoBaoData_text(
id String,
vector Array(Float32),
CONSTRAINT vec_len CHECK length(vector) = 512
) ENGINE = MergeTree ORDER BY id;
# 提取特征并填充数据库
CLIP (opens new window)是一种流行的方法,将不同形式(我们采用学术术语“模态”)的数据匹配到统一的空间中,实现高性能的跨模态检索。该模型可以对图像和文本进行编码。以下是一个示例:
import torch
import clip
from PIL import Image
# 加载CLIP模型
device = "cuda" if torch.cuda.is_available() else "cpu"
model, preprocess = clip.load("ViT-B/32", device=device)
# 加载并预处理图像
image_path = "path_to_your_image.jpg"
image = Image.open(image_path).convert("RGB")
image_input = preprocess(image).unsqueeze(0).to(device)
# 对图像进行编码
with torch.no_grad():
image_features = model.encode_image(image_input)
# 对文本进行编码
text = "Your text here"
text_input = clip.tokenize([text]).to(device)
with torch.no_grad():
text_features = model.encode_text(text_input)
# 打印图像和文本特征
print("Image features shape:", image_features.shape)
print("Text features shape:", text_features.shape)
# 将数据上传到MyScale
一旦数据被处理成嵌入向量,我们就可以将数据上传到MyScale。
# 从数据集中上传数据
client.insert("TaoBaoData_image",
data_image.to_records(index=False).tolist(),
column_names=data_image.columns.tolist())
client.insert("TaoBaoData_text",
data_text.to_records(index=False).tolist(),
column_names=data_text.columns.tolist())
# 使用余弦相似度创建向量索引
client.command("""
ALTER TABLE TaoBaoData_image
ADD VECTOR INDEX image_feature_index feature
TYPE MSTG
('metric_type=Cosine')
""")
client.command("""
ALTER TABLE TaoBaoData_text
ADD VECTOR INDEX text_feature_index feature
TYPE MSTG
('metric_type=Cosine')
""")
# 使用MyScale进行搜索
当用户输入一个问题时,我们将其转换为向量并从数据库中进行检索。这个检索过程帮助我们获取前K个产品图像及其对应的产品描述。然后,我们将这些产品描述传递给GPT模型,进一步完善推荐并提供更详细的产品介绍。此外,在最终的对话结果中,我们还向用户展示产品图像。
对问题进行编码:
question = 'Do you have any black dress for women?'
emb_query = retriever.encode(question).tolist()
搜索TaoBaoData_text数据集,返回前2个产品信息:
top_k = 2
results = client.query(f"""
SELECT id, product_text, distance(vector, {emb_query}) as dist
FROM TaoBaoData_text
ORDER BY dist LIMIT {top_k}
""")
summaries = {'id': [], 'product_text': []}
for res in results.named_results():
summaries['product_text'].append(res["product_text"])
summaries['id'].append(res["id"])
现在我们有了以下dist:
{'id':['065906','104588'],
'product_text': ['Plus Size Womens Autumn New Arrival Elegant Temperament 2019 Concealing Belly Fashionable Mid-length Lace Dress.'
'2019 Summer New Arrival High-end Asymmetrical Shoulder Strap Chic Slimming Daily V-neck Dress for Women, Trendy.']}
之后,我们可以像之前提到的那样将此列表通过OpenAI的API传递给GPT4,以下是一个示例:
system_message = f"""
Based on the user's question, we have retrieved two items with the
following information. provide recommendations for these two items based on
the product text.
{summaries}
If the user requests to see the style, please return the corresponding
product IDs.
"""
当我们有了产品ID后,我们可以搜索TaoBaoData_image数据集以获取图像,如下所示:
results = client.query(f"""
SELECT id, product_url,
FROM TaoBaoData_image
WHERE id IN {summaries['id']}
""")
65906 | 104588 |
---|---|
![]() | ![]() |
现在我们可以将这个结果返回给用户,以帮助他们做出进一步的选择和互动。
类似的流程也可以用于图像检索,例如,如果用户想要找到与图像中显示的服装相似的服装,我们可以使用图像嵌入进行检索。
# 结论
MyScale可以高效处理多模态数据,为初创公司提供了一种具有成本效益的解决方案。通过整合不同的模态和优化资源使用,MyScale增强了客户服务能力,而不会带来显著的成本。这种方法使初创公司能够有效分配资源,专注于业务的关键方面。可扩展性和成本效益对于初创公司的成功至关重要,确保可持续增长并最大化投资回报。MyScale在多模态数据处理方面的优势使初创公司能够在保持成本效益的同时扩大规模。采用MyScale使初创公司能够明智地管理资源,在竞争激烈的市场中蓬勃发展。